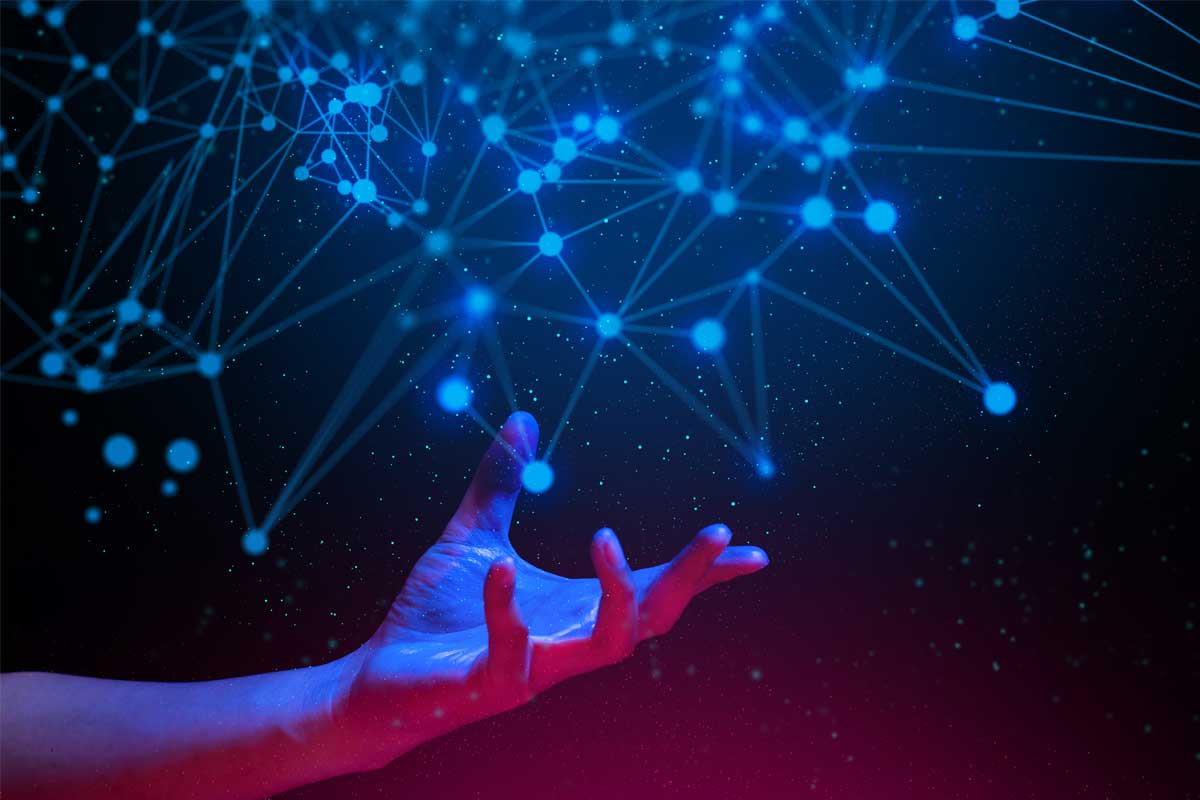
While artificial intelligence (AI) inferencing may seem seamless in daily applications, product developers and engineers on the backend know how power-hungry AI is. With each finalized product on the market came a calculated compromise to meet their energy requirements. Given how quickly AI adoption has grown over the last year1, overall energy consumption has skyrocketed, creating the need for significantly larger models and training sets. A recent study found that the AI industry could consume as much energy as a country the size of the Netherlands by 20272.
The rise of IoT has only added to the equation. Smart devices, for example, require significant energy to enable innovative use cases and lasting battery power. Efficient energy inference is an essential path forward for developers as they innovate increasingly impactful applications of AI. The bigger the network, the more you can do, right?
In this article, we’ll examine the different energy levels fueling AI compute power, their primary applications, and the future of AI inference fueled by low-power technologies.
The Energy Levels of AI Inferencing
There are three main energy levels used in AI inferencing. Each level in the hierarchy represents a trade-off between power and computational capabilities. Below, we outline those differences and how they come to life in real-world use cases:
Picojoules (pJ)
Picojoules are used for AI inferencing in ultra-low-power devices that perform simple AI tasks and face stringent power constraints. They’re also optimal for energy harvesting, the process in which energy is captured from a system’s environment and converted into usable electric power for wearables, wireless sensor networks, and more3. However, due to extremely low energy levels, picojoules may have limited computational capabilities.
Key applications in IoT at this energy level include environmental monitoring, industrial sensing, and agriculture.
Environmental monitoring involves applying AI to sensors embedded into irrigation systems, pipelines, tanks, weather stations, oceanic applications, and industrial equipment, which can detect temperature, moisture, water levels, leaks, and other physical properties. When the data is sent back to the cloud, we can track, analyze, and take further action on how resources are used and allocated.
Industrial sensing involves applying AI to temperature sensors, thermal cameras, ultrasonic sensors, photocells, inductive sensors, radar, vision cameras, and motion sensors in industrial machinery. With the sensor data, we’re able to predict and prevent machine problems before they occur.
For the agriculture industry, picojoules power levels involve applying AI to environmental sensors placed in soil or water systems. The data gathered can help with a range of issues, including crop yield prediction, selection, and management; soil compatibility classification; and water management. All aspects of smart farming contribute to feeding the growing population.
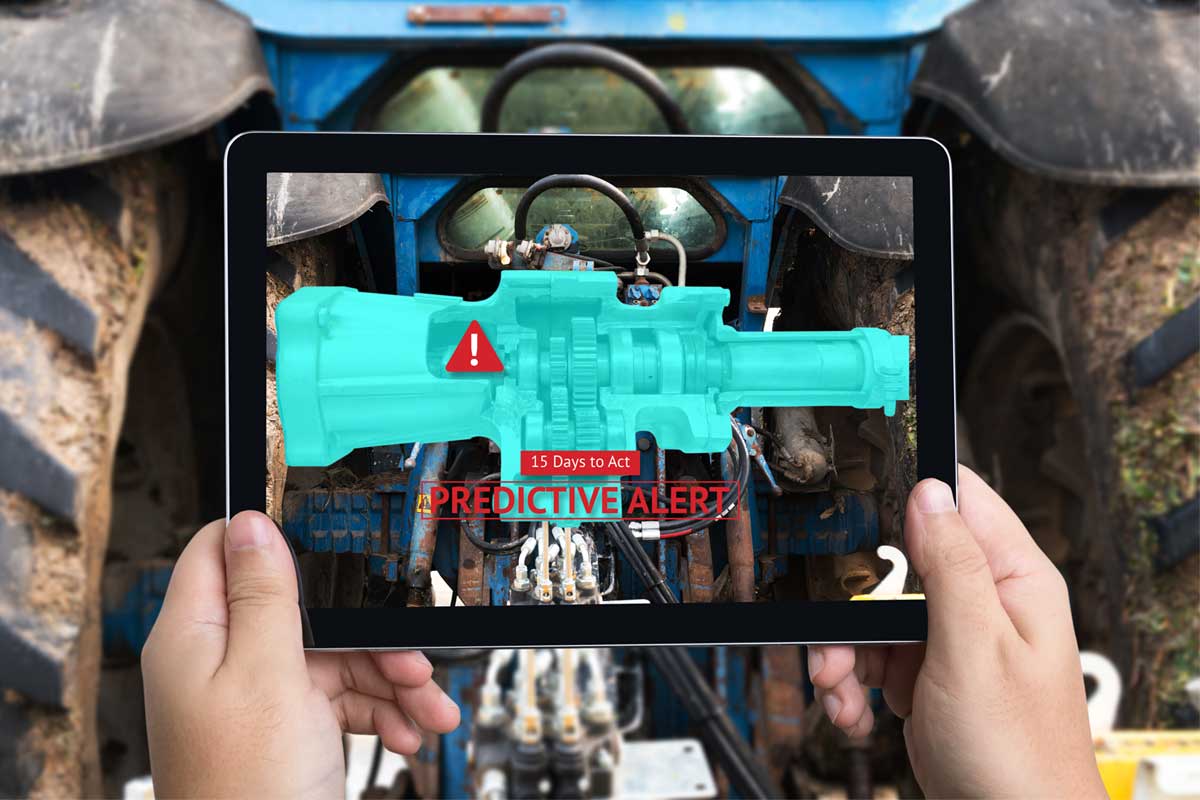
Nanojoules (nJ)
Nanojoules are often used for low-power AI applications in mobile and battery-operated devices, such as smartphones or other portable devices because they’re optimal for extending battery life and reducing the need for constant internet connection. They enable a balance between low power consumption and sufficient computational capability, so they’re suitable for a wide range of applications. While nanojoule-powered devices may be more capable than picojoule-level devices, they may still face challenges when dealing with highly complex AI computations.
Key applications in IoT at this energy level include wearables, smart homes, and remote digital health devices.
Wearables monitoring involves applying AI to gadgets — smartwatches, augmented reality technology, fitness trackers — to enhance entertainment, athletics, and other experiences. Smart home management involves applying AI to various in-home devices such as lighting, heating, speakers, etc. Machine learning picks up on the occupants’ preferences and adjusts various settings accordingly.
Remote patient monitoring is one of the fastest growing segments at this power level, which applies AI to small health wearables — pins, watches, etc. — to track patient vitals and provide personalized support, reminders about medications, and helpful insights. This use case is becoming more common as providers and patients are looking to make healthcare more efficient and result in better outcomes.
Microjoules (μJ)
Microjoules are suitable for applications where moderate power consumption is acceptable but should be minimized. They often involve more complex AI tasks, so they’re crucial for not only extending battery life but also reducing overall environmental impact. Microjoules are employed in edge computing devices where AI is done locally or in embedded systems. While still energy-efficient, microjoule-level devices may not be suitable for applications with ultra-stringent power constraints.
Key applications in IoT at this energy level include camera monitoring and automotive enhancements. Camera monitoring involves applying AI in surveillance systems, smart home cameras, motion detectors, etc. — to track and analyze humans, vehicles, attributes, and events. Automotive enhancements involve applying AI in vehicles, such as their cameras, dashboards, climate control, etc, providing new, personalized in-vehicle experiences for consumers.
How Low-Power Tech is Driving Innovation in AI Inferencing
Putting AI everywhere and incorporating it seamlessly into our daily lives is the goal. Through this transformation, power consumption will require more attention from engineers. To do more, there is a tremendous need to innovate low-power technologies that don’t impede the endpoint user’s experience. The search for the holy grail of enabling AI that consumes the least amount of power is on.
How Ambiq is Contributing
Since 2010, Ambiq, a leader of ultra low-power semiconductor solutions has helped enable over 230 million intelligent endpoint devices capable of lasting days, weeks, and even months on a single charge. Ambiq does this with the revolutionary Subthreshold Power Optimization Technology (SPOT ®) platform that provides a multi-fold in energy efficiency for smart devices. Keeping up with the increasing integration of AI within our lives, Ambiq has developed a solution that can address unique power challenges and expand the developer’s vision.
Sources:
1 The state of AI in 2023: Generative AI’s breakout year | 2023
2 Warning AI industry could use as much energy as the Netherlands | 2023
3 Three Energy-Harvesting Solutions Show Off IoT Power Possibilities | 2024
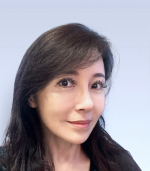
Charlene Wan