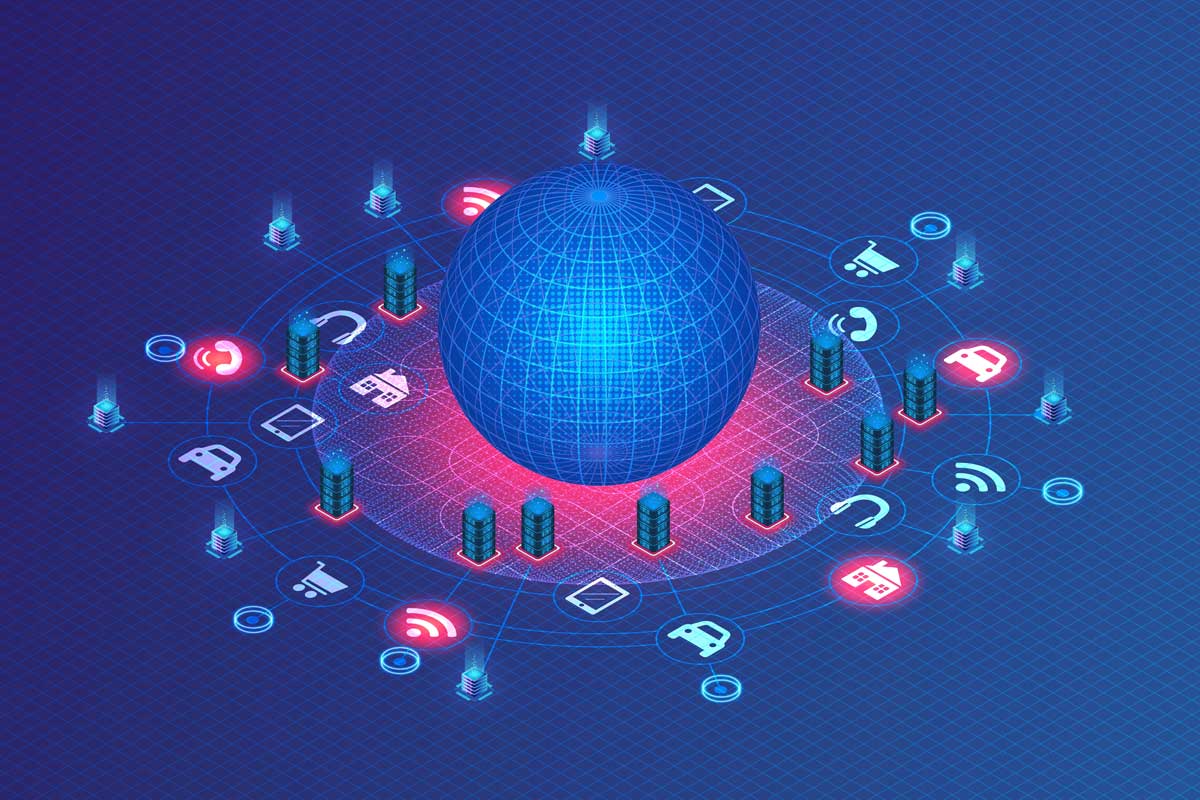
In an era dominated by interconnected technologies, integrating Artificial Intelligence (AI) and computing at the edge — connected devices is revolutionizing how we perceive and interact with smart devices. This integration, often called local device AI or edge AI computing, is an increasingly popular framework for efficiently collecting and processing data independent of the Cloud. With IOT and smart device adoption estimated to increase from 15.1 billion to 34.6 billion over the next decade1, edge AI shows promise for enhancing the power and intelligence of these devices.
Here, we’ll explore the intricacies of edge AI, including its benefits, challenges, and the promising outlook for enabling intelligent devices everywhere.
What Is Edge AI?
With an AI approach, developers deploy AI algorithms and models directly on local devices, including sensors or IoT devices, which then collect and process data locally. This convergence of on-device intelligence and machine learning (ML) capabilities provides new grounds for the potential to unlock new use cases and applications.
Benefits of Computing on a Local Device
This departure from traditional cloud-based approaches brings intelligence closer to the source of data, providing many advantages.
Enhanced Privacy and Data Security
Implementing AI on local devices empowers users with greater control over their data. Processing sensitive information locally alleviates privacy concerns and reduces the likelihood of data exposure to external networks. By moving AI to local devices, organizations responsible for maintaining the safety of their customers’ personally identifiable information (PII) are better protected.
On the devices, this data isn’t exposed to the servers of cloud service providers and other third parties, ensuring better compliance with local and international regulations around data protection. This approach mitigates the risks associated with data breaches and unauthorized access, providing a robust solution for applications requiring heightened security.
Faster Processing
The benefits of cloud-based AI come at a steep cost. Language models like GPT-3, the system upon which OpenAI built ChatGPT, require tremendous compute power to process. OpenAI had to activate traffic management strategies like a queuing system and slowing down queries to handle the surge in demand after ChatGPT’s launch2. This incident highlights how compute power is becoming a bottleneck, limiting the advancement of AI models.
Local device AI significantly reduces latency, leading to faster processing times. Applications benefit from quicker response times, enabling real-time decision-making, especially in critical scenarios such as autonomous vehicles or smart home devices. Decentralized processing also means that insights are generated in real-time with less latency than if the device had to send data to the Cloud to be processed and listen for a response.
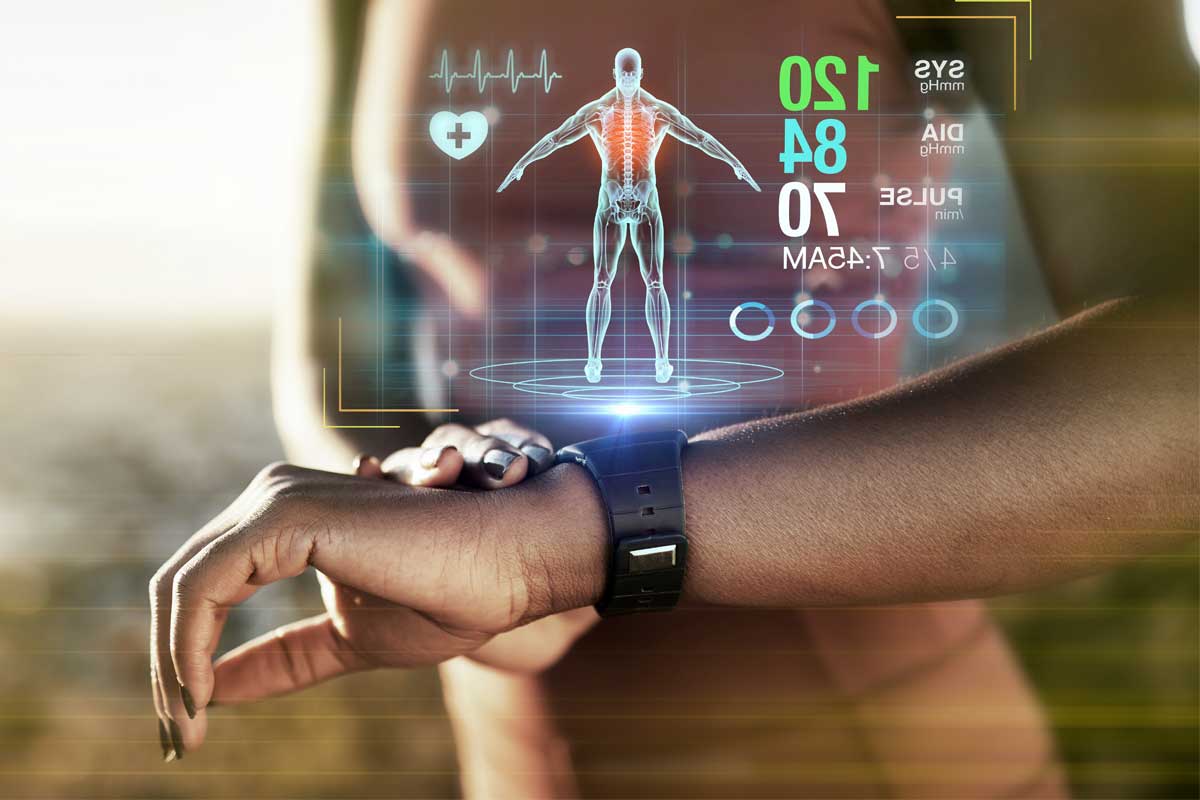
Enhanced User Experience
Reduced latency and improved processing speed provide a seamless and responsive user experience. Real-time feedback becomes possible, resulting in higher user satisfaction and engagement. Edge AI can process and analyze user data locally to create personalized experiences without relying on centralized servers3.
This leads to more responsive and tailored services, such as personalized recommendations and content delivery for cases like shopping lists, fitness apps, and meal recommendations. This type of personalized AI can more effectively captivate users and increase their engagement with content and experiences that resonate with their interests.
Reduced Dependence on the Cloud
While the Cloud has enormous power for collecting and processing data, it is susceptible to threats like hacking or outages and may not be available in areas with limited internet access4. Reducing reliance on the cloud with edge AI devices not only enhances their performance but also promotes their security from external threats. This also increases their resilience in scenarios with limited internet connectivity, particularly significant for applications in remote areas or environments with intermittent network access.
Challenges of AI on Local Devices
Some barriers exist that may limit the full potential of edge AI. These include hardware limitations, memory requirements, and power restrictions. AI models, particularly deep learning models, often demand substantial memory resources. Local devices often have constrained processing power, posing a challenge in implementing resource-intensive AI algorithms. AI computations are also power-intensive, impacting the battery life of local devices.
Mobile devices are more restricted around computing resources, memory, storage, and power consumption. As a result, on-device models need to be much smaller than their server counterparts, which can make them less powerful5. Striking a balance between functionality and resource consumption is a key challenge in designing AI-enabled devices.
The Outlook of AI on Local Devices
Despite these challenges, ongoing advancements in hardware design and optimization techniques are steadily overcoming obstacles. Technologies such as edge computing and energy-efficient processors pave the way for more efficient local device AI implementations6.
The benefits of AI and computing on local IoT-connected devices are reshaping the technological landscape, offering improved privacy, security, speed, and user experiences. While challenges exist, continuous research and innovation are overcoming these obstacles. The outlook for deploying AI on local devices appears promising, enabling a new era of intelligent devices seamlessly integrated into our daily lives.
How Ambiq is Contributing
Since 2010, Ambiq has successfully helped smart devices perform complex inferencing tasks such as AI at the edge, with their ultra-low powered semiconductor solutions. The revolutionary subthreshold power optimization technology (SPOT) platform has helped solve the power constraints smart device manufacturers run into when developing sophisticated and power-hungry features. As a result, developers can expect smooth performance with a battery life that goes for days, weeks, or months on a single charge. See more applications of Ambiq.
Sources
1 Edge AI | October 7, 2023
2 Compute power is becoming a bottleneck for developing AI. Here’s how you clear it. | March 17, 2023
3 Getting personal with on-device AI | October 11, 2023
4 Challenges of Privacy in Cloud Computing | December 2022
5 Why On-Device Machine Learning | 2024
6 Interview With Scott Hanson – Founder and CTO at Ambiq | January 4, 2024